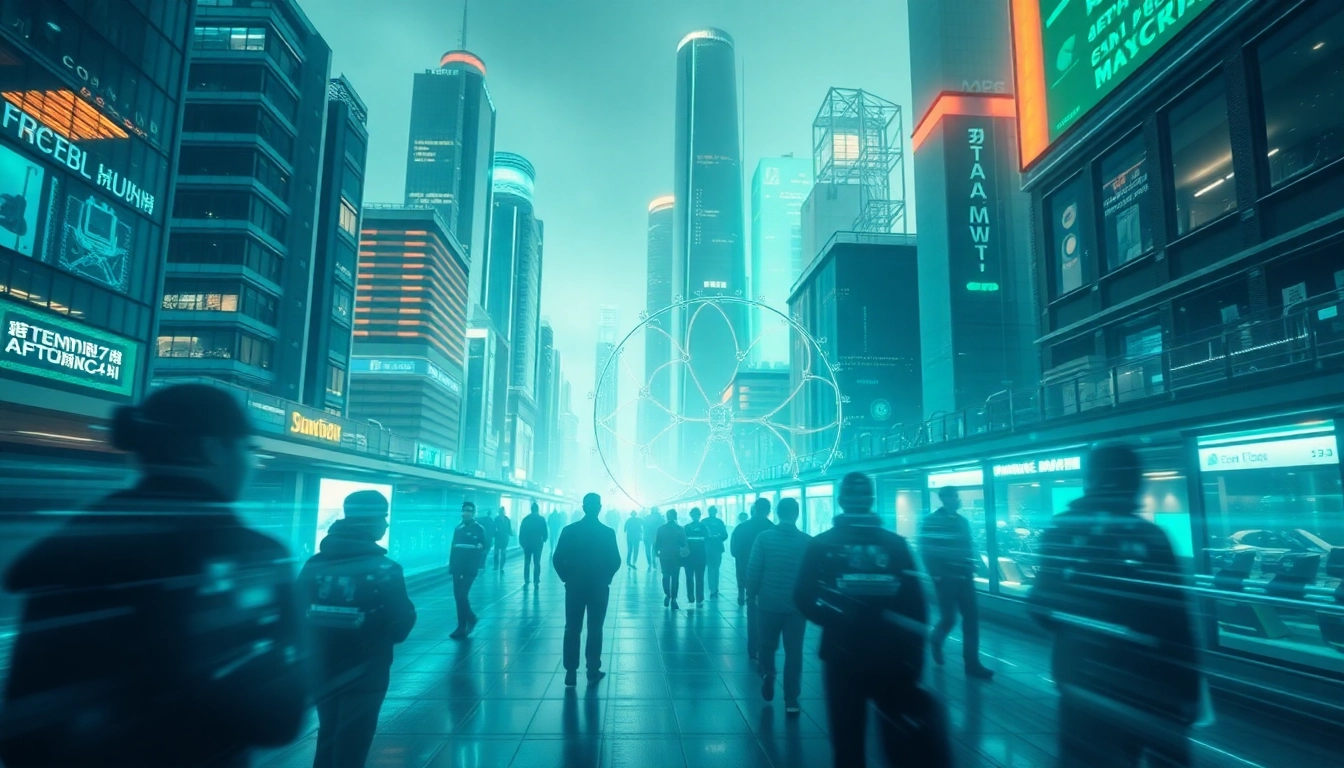
The Role of Agentic Human AI in Shaping the Future of Work and Innovation
Understanding Agentic Human AI
Definition and Concept
Agentic Human AI refers to a sophisticated subset of artificial intelligence systems capable of operating with a significant degree of autonomy. Unlike traditional AI systems that rely heavily on human intervention and supervision, agentic AI is designed to act independently, making decisions and taking actions to achieve predefined goals. This capability is grounded in advanced algorithms and machine learning techniques that allow these systems to process vast amounts of data, learn from their environments, and adapt their behaviors in real time. Such an AI can effectively manage tasks without constant guidance, thereby increasing efficiency and productivity across various applications.
The essence of agentic AI lies in its ability to function as an autonomous agent, working alongside humans rather than merely under their direction. This innovative approach not only streamlines workflows but also redefines the relationship between humans and machines in professional settings. For an in-depth exploration of this topic, you can check out Agentic Human AI as a pivotal element in the future of work.
History of Agentic AI Technology
The roots of agentic AI can be traced back to the early days of artificial intelligence research, where the primary objective was to create systems that could mimic human-like decision-making. Early AI approaches primarily relied on rule-based systems and simple algorithms lacking the sophistication necessary for independent operation. However, as machine learning and deep learning technologies advanced, significant breakthroughs in data processing and algorithm efficiency have paved the way for the development of agentic AI.
One foundational milestone was the introduction of reinforcement learning in the early 2000s, enabling machines to learn optimal behaviors through trial and error. This model has been instrumental in developing systems that autonomously make decisions based on environmental feedback. Over the past decade, the integration of neural networks and natural language processing has further enriched the capabilities of agentic AI, allowing these systems to operate more effectively in complex and dynamic environments.
Core Features and Capabilities
Agentic Human AI systems are characterized by several key features that enable their autonomous functionalities:
- Autonomy: The primary feature of agentic AI is its ability to operate independently without requiring continuous human input. This self-sufficiency is essential for enhancing operational efficiency.
- Contextual Awareness: These systems can understand and interpret their environment, adjusting their behaviors based on the context in which they operate. This feature makes agentic AI adaptable to varying scenarios and requirements.
- Learning and Adaptation: Agentic AI employs advanced machine learning algorithms that allow for continual learning from experience, enabling it to improve its performance over time. This aspect of learning equips the AI with the ability to foresee future situations and adjust strategies accordingly.
- Goal-Oriented Actions: Unlike conventional AI, which may focus on singular tasks, agentic AI is designed to pursue broader objectives, aligning its actions with specific goals set by human operators or organizational mandates.
- Complex Decision-Making: These AI systems utilize sophisticated reasoning techniques to evaluate various possible outcomes and make decisions based on complex data analysis, significantly enhancing problem-solving capabilities.
Applications of Agentic Human AI
Industries Leveraging Agentic AI
Agentic Human AI technologies are being adopted across diverse industries due to their potential to streamline operations and enhance productivity. Some prime sectors include:
- Healthcare: In medical settings, agentic AI systems assist in diagnostics, treatment planning, and even robotic-assisted surgeries, improving patient outcomes while minimizing human error.
- Finance: Financial institutions leverage agentic AI for automated trading, fraud detection, and risk management, which helps optimize investment strategies and safeguard against fraudulent activities.
- Manufacturing: In manufacturing, agentic AI enables predictive maintenance, supply chain optimization, and quality control, leading to reduced operational costs and enhanced reliability.
- Customer Service: AI-driven chatbots and virtual assistants use agentic capabilities to engage with customers, provide support, and analyze feedback to improve service quality.
- Logistics: The logistics sector benefits from agentic AI through intelligent routing, inventory management, and demand forecasting, accommodating fluctuations in supply and demand effectively.
Real-World Use Cases
To illustrate the practical applications of agentic AI, several real-world use cases demonstrate its transformative potential:
- Autonomous Vehicles: Companies like Waymo and Tesla are employing agentic AI to develop self-driving cars capable of navigating various road conditions and making independent traffic decisions.
- Supply Chain Management: Advanced analytics powered by agentic AI is deployed by companies like Amazon to optimize their supply chains, anticipate shipping delays, and manage inventories autonomously.
- Healthcare Diagnostics: IBM Watson Health exemplifies the use of agentic AI in analyzing medical data to provide diagnostic suggestions and treatment recommendations with minimal human intervention.
- Smart Manufacturing: Businesses such as Siemens are utilizing agentic AI to enable machines that monitor production processes, predict failures, and implement corrective actions on the fly.
- Personalized Marketing: Retailers like Netflix use agentic AI for recommending products and content based on individual user preferences, behaviors, and viewing histories by autonomously adjusting marketing strategies.
Benefits of Adoption
The proliferation of agentic AI technologies offers several advantages to organizations willing to adopt them:
- Enhanced Efficiency: By minimizing human intervention, agentic AI accelerates workflows, allowing employees to focus on more strategic tasks that require human creativity and critical thinking.
- Cost Reduction: Reduced dependence on personnel for routine decision-making results in lower operational costs, contributing directly to improved profitability.
- Improved Accuracy: The inherent capabilities of agentic AI to analyze vast datasets lead to more precise decision-making, reducing errors and increasing overall effectiveness.
- Scalability: As businesses grow, so do their data processing needs. Agentic AI can be easily scaled to accommodate these demands without a proportional increase in workforce.
- Innovation Facilitation: Organizations tapping into agentic AI often find it easier to experiment with new ideas, creating an environment conducive to innovation and adaptation.
The Future of Work with Agentic Human AI
Impact on Job Roles and Skillsets
The integration of agentic AI into the workplace is poised to significantly alter job roles and the skill sets required across various industries. It is imperative for professionals to adapt to changes that may result in job displacement in some areas while creating new opportunities in others. Here are the expected changes:
- Shifting Job Descriptions: As AI takes over routine tasks, job descriptions will evolve, focusing more on strategic oversight, innovation, and complex problem-solving rather than repetitive tasks.
- Need for Tech-Savvy Workers: A workforce that is comfortable with AI tools and understands their functionalities will be in high demand. Upskilling through training in data analysis and AI literacy will become essential.
- Human-AI Collaboration: New roles will emerge focused on fostering collaboration between AI systems and human teams, where professionals will interpret AI-generated insights and make nuanced decisions.
- Resilience and Flexibility: Workers will need to cultivate adaptability, resilience, and a continuous learning mindset to keep pace with developing technologies and changing workplace dynamics.
Change Management Strategies
Successfully integrating agentic AI into an organization requires strategically managing the change that accompanies such technological advancements. Here are effective strategies:
- Stakeholder Engagement: Involving all stakeholders from the outset fosters a sense of ownership and eases apprehensions associated with change.
- Clear Communication: Transparent communication ensures that employees understand the rationale behind implementing agentic AI and how it impacts their roles.
- Training and Development: Providing opportunities for training and upskilling helps employees adjust to new technologies and enhances their confidence in working alongside AI.
- Pilot Programs: Initiating pilot projects allows organizations to test agentic AI solutions on a smaller scale, gauging effectiveness and gathering feedback before full-scale adoption.
Preparing for Integration
Organizations interested in adopting agentic AI should consider several preparatory steps to ensure a smooth transition:
- Assess Existing Infrastructure: Evaluating current technological conditions ensures that systems are capable of supporting the implementation of agentic AI solutions.
- Identify Use Cases: Clear identification of specific business problems that agentic AI can address facilitates a more targeted and effective deployment.
- Measure Current Performance: Establishing baseline performance metrics enables organizations to assess the impact of AI integration effectively.
- Develop a Roadmap: A comprehensive roadmap should outline the stages of implementation, resources needed, and timelines to guide the integration process.
Ethical Considerations and Challenges
Regulatory Frameworks
The rise of agentic AI raises significant regulatory challenges that stakeholders must address. Existing regulations may not adequately cover the complexities introduced by autonomous systems, necessitating the formation of new frameworks that consider safety, liability, and ethical standards. Policymakers must work alongside AI developers and business leaders to establish rules that govern the deployment and operation of agentic AI technologies, ensuring that ethical practices are embedded in their use.
Transparency and Trust Issues
Transparency in AI operations is critical to fostering trust among users and stakeholders. Agentic AI systems must provide insight into their decision-making processes, enabling users to understand how outcomes are derived. Mechanisms should be in place to audit AI activities regularly and reassess them to ensure adherence to ethical standards and operational accuracy.
Mitigating Risks of Autonomy
The inherent autonomy of agentic AI presents risks that organizations need to proactively address. Potential issues include decision-making that may not align with ethical or legal standards, data privacy concerns, and the susceptibility to biases inherent in AI training data. To mitigate these risks:
- Implement Robust Oversight: Supervision frameworks should be established to monitor AI activities, evaluate performance against ethical benchmarks, and intervene when necessary.
- Develop Comprehensive Training Protocols: Efforts should focus on ensuring that AI systems train on diverse datasets to minimize bias and enhance fairness in decision-making.
- Encourage Continuous Feedback Loops: Incorporating user feedback into AI systems can help refine behaviors, align actions with user expectations, and enhance overall efficacy.
Getting Started with Agentic Human AI
Implementing Agentic AI Solutions
Successfully implementing agentic AI solutions requires a structured approach. Here are key steps organizations should consider:
- Define Clear Objectives: Organizations need to articulate specific goals that agentic AI will achieve, tailoring solutions to meet these objectives effectively.
- Choose the Right Technology Partner: Selecting an experienced vendor that aligns with the organizational goals and provides suitable technology options is crucial.
- Start Small: Pilot projects should be initiated to test the feasibility of solutions before scaling them across the organization.
- Establish Evaluation Metrics: Define success metrics upfront to evaluate performance and impact post-implementation effectively.
Measuring Success and Performance Metrics
To gauge the effectiveness of agentic AI initiatives, organizations should develop and track performance metrics. Key indicators may include:
- Operational Efficiency: Metrics related to the time and resource savings realized through the automation of tasks.
- Quality of Decisions: Measuring the accuracy and effectiveness of decisions made by agentic AI compared to previous human-led decision-making.
- User Satisfaction: Gathering feedback from users interacting with agentic AI systems to assess their experiences and satisfaction with performance.
- Innovation Rate: Tracking the rate and quality of innovations stemming from new possibilities presented by the integration of agentic AI.
Continual Learning and Adaptation
Agentic AI systems should be designed to facilitate continual learning, adapting to changing operational landscapes and new data inputs. Organizations can leverage feedback loops to refine AI behaviors and ensure that systems remain relevant and effective. Businesses should invest in training programs that empower employees to engage with agentic AI actively, providing ongoing education and opportunities to enhance collaboration between human workers and AI systems.