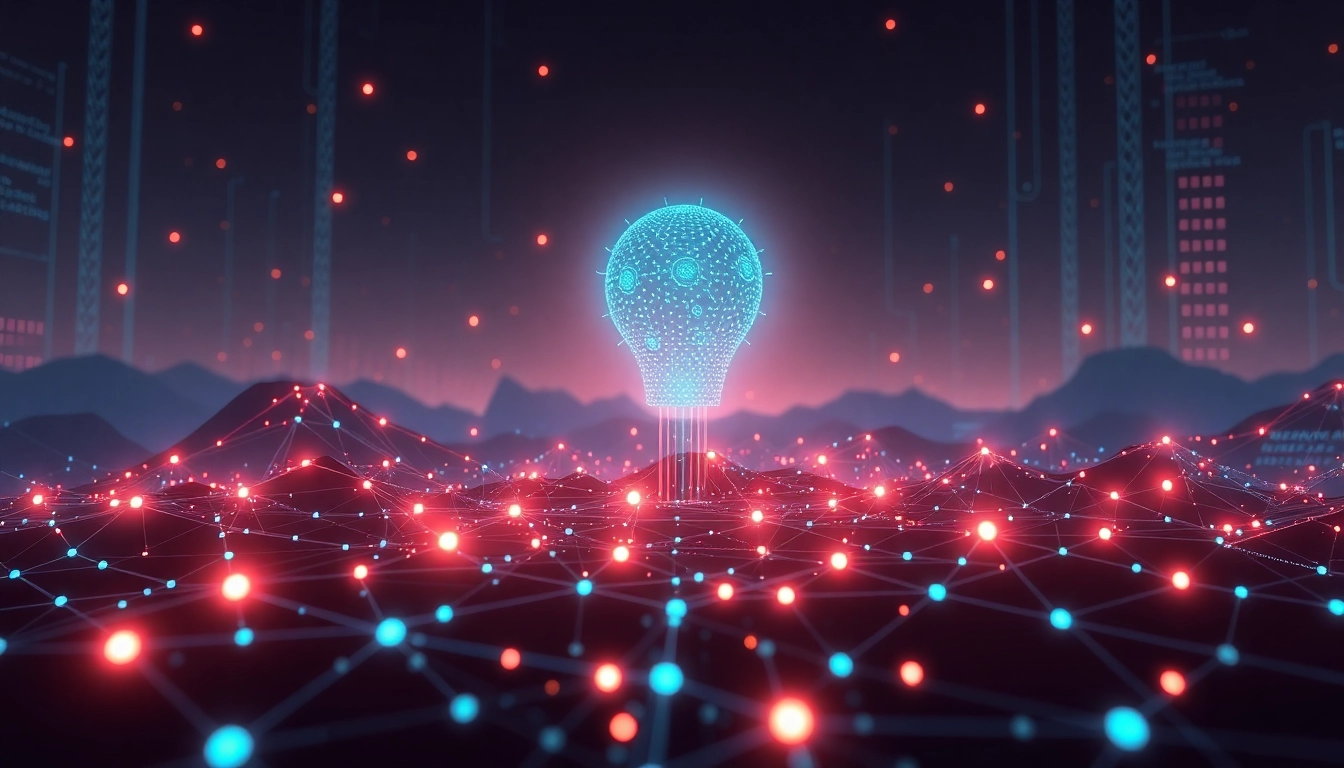
The Rise of Agentic AI: Understanding Autonomous Intelligence in Today’s Technology
Introduction to Agentic AI
In the evolving landscape of artificial intelligence, Agentic AI emerges as a vital player, poised to redefine how we interact with machines and technology. Unlike traditional AI systems, which often require constant human oversight and intervention, agentic AI systems are capable of making autonomous decisions, learning from their environments, and executing complex tasks without human input. This shift towards greater autonomy brings forth not only exciting possibilities but also considerable challenges that we must navigate in its integration into society.
Defining Agentic AI and Its Importance
Agentic AI refers to artificial intelligence systems that can operate independently, analyze various situations, and make informed decisions to achieve specific goals. This type of AI processes vast amounts of data to autonomously plan actions, solve multi-step problems, and adapt to changing circumstances—qualities that allow organizations to streamline operations, reduce costs, and enhance productivity. The importance of agentic AI can be attributed to its potential to transform various aspects of business and everyday life, from automating mundane tasks to enabling advanced analytics and real-time decision-making.
The Evolution of Autonomous Technologies
The concept of autonomous systems is not new; however, recent advancements in machine learning, data processing, and computational power have propelled the capabilities of agentic AI. Historical milestones such as the development of expert systems in the 1980s and the emergence of machine learning applications in the 2000s laid the groundwork for today’s sophisticated agentic systems. As we move into an era defined by rapid technological change, these systems are increasingly being integrated into diverse sectors including finance, healthcare, logistics, and customer service.
Key Characteristics of Agentic AI
Agentic AI is defined by several distinguishing characteristics that set it apart from conventional AI systems:
- Autonomy: Agentic AI can operate independently, evaluating situations without requiring human guidance.
- Adaptability: These systems learn and adapt to new data and environments in real time, improving their effectiveness over time.
- Self-Optimization: They can iteratively improve their algorithms based on feedback, enhancing decision-making processes.
- Goal-Oriented Behavior: Agentic AI focuses on specific objectives, performing tasks efficiently to achieve those goals.
How Agentic AI Works
Core Algorithms Behind Decision Making
At the heart of agentic AI lies a complex framework of algorithms that facilitate decision-making. These algorithms often include a combination of supervised and unsupervised learning methods, neural networks, and reinforcement learning techniques. For example, agentic AI systems may use deep learning to analyze patterns and make predictions based on historical data. The capability to process and learn from vast datasets is fundamental, enabling these systems to identify trends and optimize their performance over time.
The Role of Machine Learning
Machine learning is a cornerstone of agentic AI, allowing systems to learn from experiences and improve their decisions without explicit programming. In agentic AI, machine learning algorithms are employed to train the system on past data, enabling it to understand the nuances of the scenarios it will face. For instance, in a financial application, an agentic AI may analyze stock market data to make trades, learning from the outcomes of its previous decisions to refine its trading strategies continuously.
Real-Time Adaptability in Dynamic Environments
One of the most compelling features of agentic AI is its ability to adapt to dynamic environments. Unlike static systems that rely on pre-defined rules, agentic AI utilizes real-time data to make decisions that reflect current conditions. This adaptability is particularly valuable in industries such as logistics where factors such as traffic, weather, and demand fluctuations can significantly impact operations. By continuously updating its models and strategies, agentic AI can optimize resource allocation, enhance efficiency, and reduce operational costs.
Applications of Agentic AI
Use Cases in Different Industries
Agentic AI is being applied across a multitude of industries, demonstrating its versatility and effectiveness:
- Healthcare: In healthcare, agentic AI systems can analyze patient data to assist in diagnostics, personalize treatment plans, and improve patient management.
- Finance: Financial institutions use agentic AI for fraud detection, risk assessment, and financial forecasting, automating complex decision-making processes.
- Logistics: Agentic AI optimizes supply chain management by predicting demand, managing inventories, and enhancing delivery routes.
- Customer Service: Businesses leverage agentic AI for chatbots and virtual assistants that resolve customer queries autonomously, improving user experience.
Benefits of Implementing Agentic AI Solutions
The implementation of agentic AI leads to numerous benefits:
- Increased Efficiency: By automating routine tasks, organizations can free up human workers to focus on high-value activities.
- Cost Reduction: Streamlining operations through agentic AI reduces operational costs and resource usage.
- Improved Accuracy: The ability to analyze large datasets enhances decision-making accuracy compared to traditional methods.
- Enhanced Customer Experience: With faster and more efficient service delivery, businesses improve customer satisfaction and loyalty.
Challenges and Considerations
Despite its advantages, implementing agentic AI solutions comes with challenges that organizations must navigate:
- Data Quality: Effective agentic AI systems require high-quality and diverse datasets to function optimally. Organizations must ensure their data is clean, relevant, and representative.
- Integration Issues: Integrating agentic AI with existing systems can be complex, requiring careful planning and execution.
- Ethical Concerns: The autonomous decision-making capabilities of agentic AI may pose ethical dilemmas, especially in areas like healthcare and law enforcement.
Comparing Agentic AI and Generative AI
Distinct Functions and Capabilities
While both agentic AI and generative AI represent significant advancements in the AI domain, they serve distinct purposes. Agentic AI is focused on achieving specific outcomes through decision-making and actions, while generative AI is centered on creating new content such as text, images, or designs based on input parameters. This difference marks a fundamental shift in the capabilities and applications of AI technologies.
Integration with Existing Systems
Agentic AI and generative AI also differ when it comes to integration with existing systems. Agentic AI often requires direct interfacing with operational systems to execute actions, necessitating a seamless coordination layer. Generative AI, on the other hand, can function independently or in collaboration with creative workflows, thus allowing greater flexibility in its deployment.
Use Cases for Each Type of AI
The use cases for agentic AI and generative AI reflect their respective focuses:
- Agentic AI: Common applications include analytics, autonomous vehicles, predictive maintenance, and real-time optimization in logistics.
- Generative AI: Examples include content generation for social media, automated report writing, and creative design processes.
The Future of Agentic AI
Trends and Predictions for 2025
As we look ahead, the following trends are expected to shape the future of agentic AI in the coming years:
- Increased Adoption: More businesses across various sectors will adopt agentic AI technologies to enhance productivity and streamline operations.
- Enhanced Collaboration: The collaboration between human workers and agentic AI will redefine job roles, requiring new skills and training.
- Greater Regulation: As these technologies become more pervasive, regulatory frameworks will likely emerge to manage their ethical and societal implications.
Impact on Work and Everyday Life
The integration of agentic AI into daily life and workplaces is set to transform how we approach tasks, communication, and decision-making. Organizations will benefit from increased efficiency, while employees will experience changes in their roles as they collaborate with intelligent systems. The impact on everyday life may include smarter home devices and improved healthcare delivery.
Potential Ethical Concerns
With great power comes great responsibility; thus, the deployment of agentic AI raises several ethical concerns that must be addressed:
- Accountability: Determining responsibility for the actions taken by autonomous systems can be complex and requires clear guidelines.
- Bias and Fairness: Agentic AI systems must be designed to prevent biases that can lead to unfair treatment of individuals or groups.
- Privacy: The use of extensive data raises concerns about privacy and data protection that organizations must navigate cautiously.