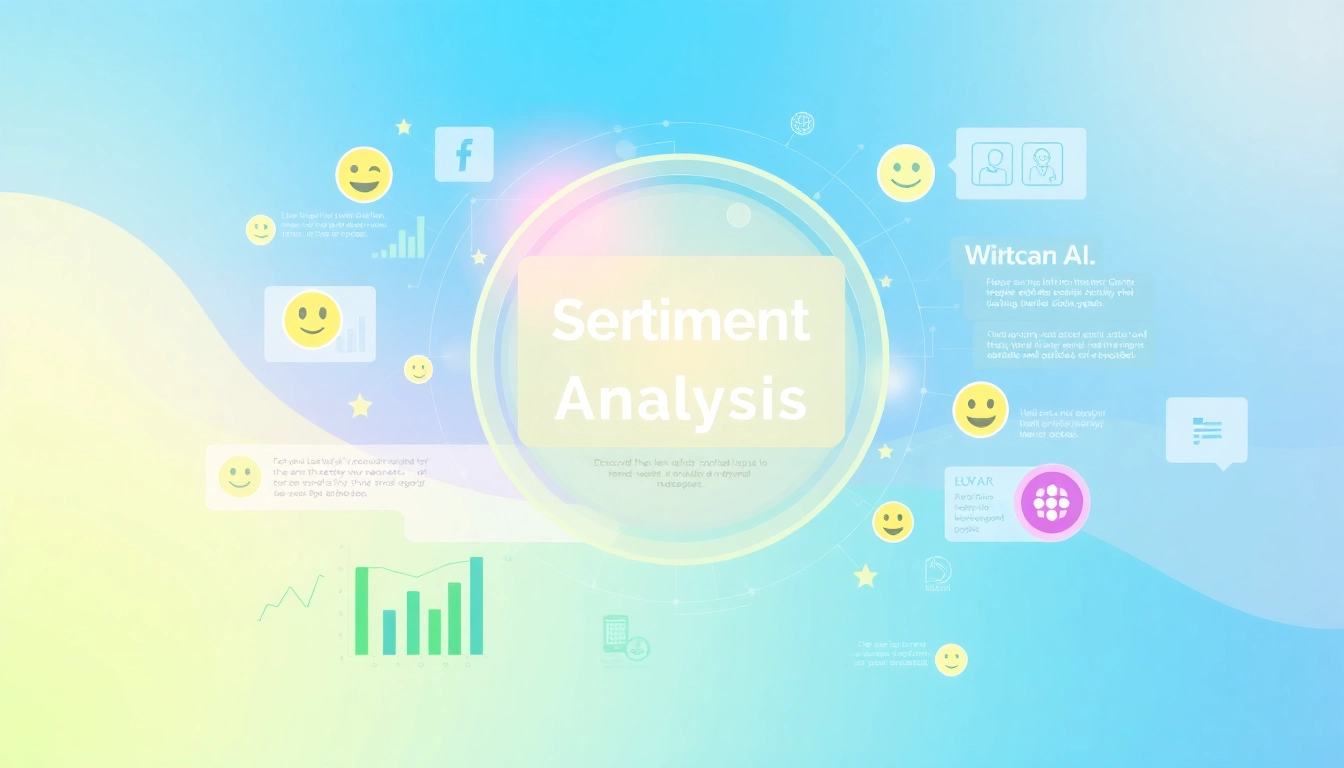
AI Sentiment Analysis: Unlocking Emotional Insights for Better Business Decisions
Understanding AI Sentiment Analysis
What is Sentiment Analysis?
Sentiment analysis refers to the use of natural language processing (NLP) and machine learning techniques to analyze textual data and determine the emotional tone behind a series of words. It serves as a powerful tool for businesses and researchers alike, using algorithms to classify text as positive, negative, or neutral. By leveraging this analysis, organizations can gain valuable insights into public opinion, consumer behavior, and social sentiment, which aid in decision-making processes.
This analytical technique sifts through vast amounts of unstructured data, such as reviews, social media comments, and surveys, providing stakeholders with a clearer understanding of customer attitudes and feelings.AI sentiment analysis allows companies to respond strategically to customer feedback, improving engagement and outcomes.
Importance of Sentiment Analysis in Today’s Market
In an age where customer feedback can be gathered in real-time from multiple digital platforms, sentiment analysis emerges as a critical resource. The insights gained from analyzing consumer sentiment can lead to actionable business strategies. Companies implementing sentiment analysis can better understand trends, preferences, and areas needing improvement.
Businesses that utilize sentiment analysis have reported enhanced customer satisfaction, increased loyalty, and the ability to tailor products and services to meet evolving consumer demands. As competition intensifies, accurately gauging public sentiment can provide a crucial edge in developing marketing strategies, managing brand reputation, and facilitating product development.
How AI Enhances Sentiment Analysis
Artificial intelligence significantly enhances the efficacy of traditional sentiment analysis methods. By employing sophisticated algorithms, AI can process large datasets far more efficiently and accurately than human analysts. AI tools harness NLP to comprehend context, sarcasm, and emotional subtleties often lost in basic sentiment classification.
The integration of AI allows for continuous learning: as more data is analyzed, algorithms become sharper in accuracy. This iterative learning is essential for adapting to the changing language used across social media and other platforms, facilitating real-time insights that are crucial for timely decision-making.
Key Techniques in AI Sentiment Analysis
Natural Language Processing (NLP) Explained
Natural Language Processing is the backbone of AI sentiment analysis. It encompasses a range of techniques that enable machines to understand, interpret, and generate human language in a valuable way. Key techniques in NLP include tokenization, sentiment scoring, lemmatization, and part-of-speech tagging.
Tokenization breaks down text into individual words or phrases, while lemmatization simplifies these words to their base forms. Sentiment scoring assigns numerical values to emotions expressed in the text, making it easier to quantify and analyze sentiment overall.
Through machine learning, NLP systems can train on vast datasets, iteratively improving their understanding and classification capabilities, making them crucial for effective sentiment analysis.
Machine Learning Algorithms for Sentiment Detection
Various machine learning algorithms are employed in sentiment analysis, each with its strengths and weaknesses. Commonly used models include:
- Naïve Bayes Classifier: This probabilistic model excels in textual data and performs efficiently with a relatively small feature set.
- Support Vector Machines (SVM): SVM is effective for high-dimensional spaces, making it a robust choice for feature-rich datasets.
- Deep Learning Techniques: Neural networks, particularly recurrent neural networks (RNNs) and convolutional neural networks (CNNs), have gained traction for sentiment classification due to their ability to capture complex patterns in data.
Each algorithm’s choice ultimately hinges on the specific needs of the analysis, including volume, complexity, and the nuances of the dataset being evaluated.
Utilizing Big Data for Enhanced Analysis
Big data plays a pivotal role in AI sentiment analysis by providing access to large volumes of varied data sources. Social media, customer feedback, and online reviews generate vast amounts of data that, when harnessed correctly, can yield deep insights into consumer attitudes.
By employing big data analytics, companies can identify trends, spot emerging issues before they escalate, and gauge overall brand health. This real-time analysis is crucial, especially for businesses competing in fast-moving industries, ensuring they remain responsive to consumer needs and market dynamics.
Applications of AI Sentiment Analysis
In Marketing Strategies
Marketing teams increasingly rely on AI sentiment analysis to devise targeted strategies. By analyzing consumer sentiment towards specific campaigns or products, businesses can fine-tune their messaging and identify which elements resonate with their audience.
Moreover, sentiment analysis enables marketers to track the effectiveness of advertising campaigns in real-time, allowing for an agile marketing approach that can adapt based on consumer reactions.
Customer Service Improvements
Customer service departments utilize sentiment analysis to enhance their engagement efforts. By gauging customer sentiment in interactions across various channels (like email, chat, and social media), businesses can identify unhappy customers and intervene proactively.
Sentiment analysis systems can alert customer service representatives to potentially negative experiences, allowing them to address issues before exacerbating customer dissatisfaction, ultimately leading to improved retention rates.
Monitoring Brand Reputation
For businesses, maintaining a positive brand reputation is paramount. AI sentiment analysis provides organizations with the tools to monitor brand sentiments expressed in the digital landscape through social listening strategies.
By analyzing how consumers perceive their brand versus competitors, companies can respond to negative sentiment promptly and strategize on improvements. This real-time monitoring can mitigate crises before they escalate, safeguarding brand integrity.
Best AI Tools for Sentiment Analysis
Top Free and Paid Tools Reviewed
The market offers a plethora of AI sentiment analysis tools catering to different needs. Some of the top tools include:
- IBM Watson Natural Language Understanding: A robust tool that provides deep insights through machine learning and natural language processing.
- Lexalytics: This platform offers advanced analytics capabilities and can process and analyze large volumes of textual data.
- MonkeyLearn: An accessible tool that requires no coding skills, making it great for businesses wanting to dive into sentiment analysis.
Each of these tools brings unique features, and the choice depends on specific organizational requirements and budget considerations.
Comparing Features and Benefits
When selecting sentiment analysis tools, organizations should consider features such as multilingual support, integration capabilities, and ease of use. For instance, while some tools excel in English best practices, others may offer better multilingual sentiments analysis which is essential for global brands.
Another key factor is real-time analysis capabilities. Organizations should explore tools that can process and interpret data live, allowing for swift responses to evolving sentiments.
User Experiences and Case Studies
Real-world use cases can provide insight into assisting companies in understanding customer sentiment. For instance, a retail brand observing sentiment analysis through customer interactions discovered that negative feedback about online delivery times significantly impacted customer satisfaction scores.
By implementing changes based on sentiment insights, such as improving logistics and communication about shipping updates, the brand not only mitigated negative sentiment but enhanced overall customer satisfaction.
Future Trends in AI Sentiment Analysis
The Role of Generative AI
Generative AI is poised to revolutionize sentiment analysis further by enhancing the context and nuance captured through textual data. By generating responses that reflect emotional tones, generative AI can assist in simulating conversations that capture genuine sentiments.
This technology can also aid in content generation, helping brands to respond to customer feedback in ways that resonate emotionally, extending the reach of their sentiment analysis beyond mere recognition into meaningful engagement.
Integration with Other AI Technologies
As the landscape of artificial intelligence evolves, we can expect AI sentiment analysis to integrate more seamlessly with other AI technologies. This includes enhanced machine learning algorithms that allow for more precise classifications, and improvements in data analytics that can combine sentiment data with behavioral analytics for more comprehensive insights.
The convergence of these technologies will create richer data landscapes where emotional analytics can be combined with demographic and behavioral data, allowing businesses to develop a holistic view of consumer sentiment and behaviors.
Emerging Market Predictions
The future of AI sentiment analysis is bright, with market demand steadily increasing as businesses recognize its value. Industries such as e-commerce, tourism, and finance are expected to lead in adoption as they become more customer-centric.
Additionally, there is a growing recognition of the need for ethical AI practices in sentiment analysis to ensure data privacy and minimize bias. Companies addressing these concerns proactively may not only foster goodwill but also gain competitive advantages in increasingly crowded markets.